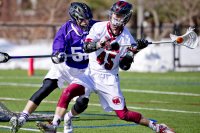
In milliseconds, our brains take the information that meets our eyes and categorize it into everyday scenes like offices or kitchens, associated with memories, emotions, and applications for our daily lives.
Neuroscientists who study such scene categorization run into a challenge, says Assistant Professor of Neuroscience Michelle Greene. It’s tough to measure how what Greene calls “features” of a scene — such as colors, textures, or the words we associate with a scene — contribute to categorization, especially since each feature affects the others.
So Greene and a colleague set out to mathematically model the role each feature plays in scene categorization over time, part of a research project funded by the National Science Foundation. In doing so, they found that complex features like function contribute to categorization as early in the process as simple ones, like color.
Greene’s resulting paper, “From Pixels to Scene Categories: Unique and Early Contributions of Functional and Visual Features,” co-authored with Colgate University’s Bruce C. Hansen, won best paper at the Conference on Cognitive Computational Neuroscience in September.
Hansen and Greene, who uses machine learning to study visual perception, identified 11 features that contribute to scene categorization. Some of them are “low-level” features, meaning a computer could recognize them, Greene says. These include colors, textures, and edges.
Some are “high-level” features, meaning only humans can label them. These include specific objects, like a blender in a kitchen or a computer monitor in an office, as well as function, which refers to what a person might do in a scene, such as sleeping in a bedroom.
Human brains process these features in conjunction with each other to comprehend scenes — in our minds, at the instant of recognizing a scene, an individual feature can’t be isolated.
“If you change the geometry of the room, that’s also going to change the low-level features of the room,” Greene says. “If you change the low-level features, it’s going to change the high-level features.”
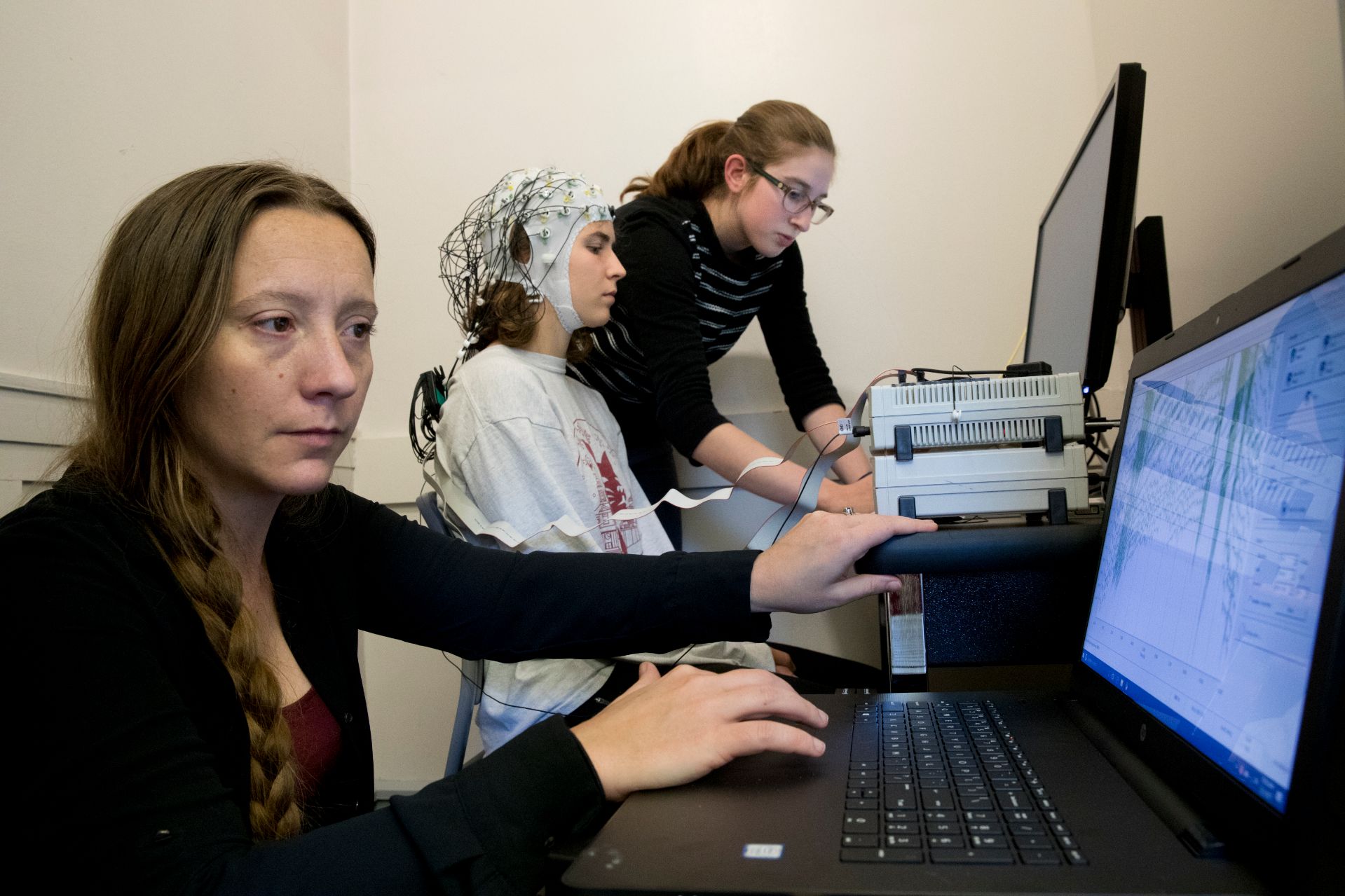
In December 2017, Assistant Professor of Neuroscience Michelle Greene works with Hanna De Bruyn ’18 to run an EEG test with Katie Hartnett ’18. (Phyllis Graber Jensen/Bates College)
Greene and Hansen found ways to measure the effects of various features individually. For example, to measure functions, they took the American Time Use Survey, which asks people how they spend their time, and associated the answers — watching TV, cooking, working — with different scenes, like a living room, a kitchen, and an office.
Once they had a way to track individual features, they gathered a selection of thousands of images and, using both computer coding and human categorization through Amazon’s Mechanical Turk tool, associated high- and low-level features with each scene.
With all that data in hand, they used a technique in linear algebra called whitening transformation to orthogonalize, or parse out, each individual feature so they could study it independently from the others.
“We put all of these features together in a nice big matrix and de-correlated them,” Greene says. “Here’s color by itself, here’s edges by itself, objects by itself, so on and so forth.”
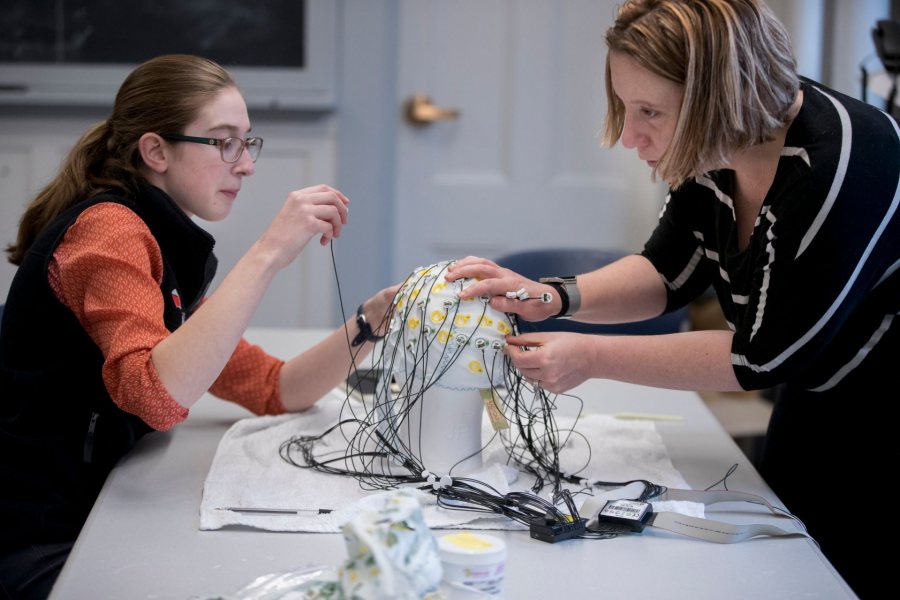
In March, Michelle Greene and Hanna De Bruyn ’18 prepare to give an EEG test for De Bruyn’s senior thesis. Greene works extensively with students on her research. (Phyllis Graber Jensen/Bates College)
Greene and Hansen then compared the results of their model to human brain activity using EEG tests.
“We can get, in a millisecond-by-millisecond way, the extent to which similarity in the EEG patterns tracks similarity with regard to any of these orthogonalized features,” Greene says.
Greene originally thought that the brain would perceive low-level features like color and texture first, then bring in high-level features in order to identify the scene. Instead, she found that high-level features are involved in visual processing early on.
Greene will delve deeper into how individual features affect visual perception, studying how manipulating one feature in an image affects how we process the image. She’ll also see if a brain processes images differently based on whether its owner is told to focus on a specific feature, such as color.
Greene works extensively with Bates students on these questions — she says the students get to learn computer programming and how to run EEG tests, and Greene herself gets fresh perspectives on her work.
Sometimes, students “see that this obvious assumption is an assumption, and we should test it,” she says.